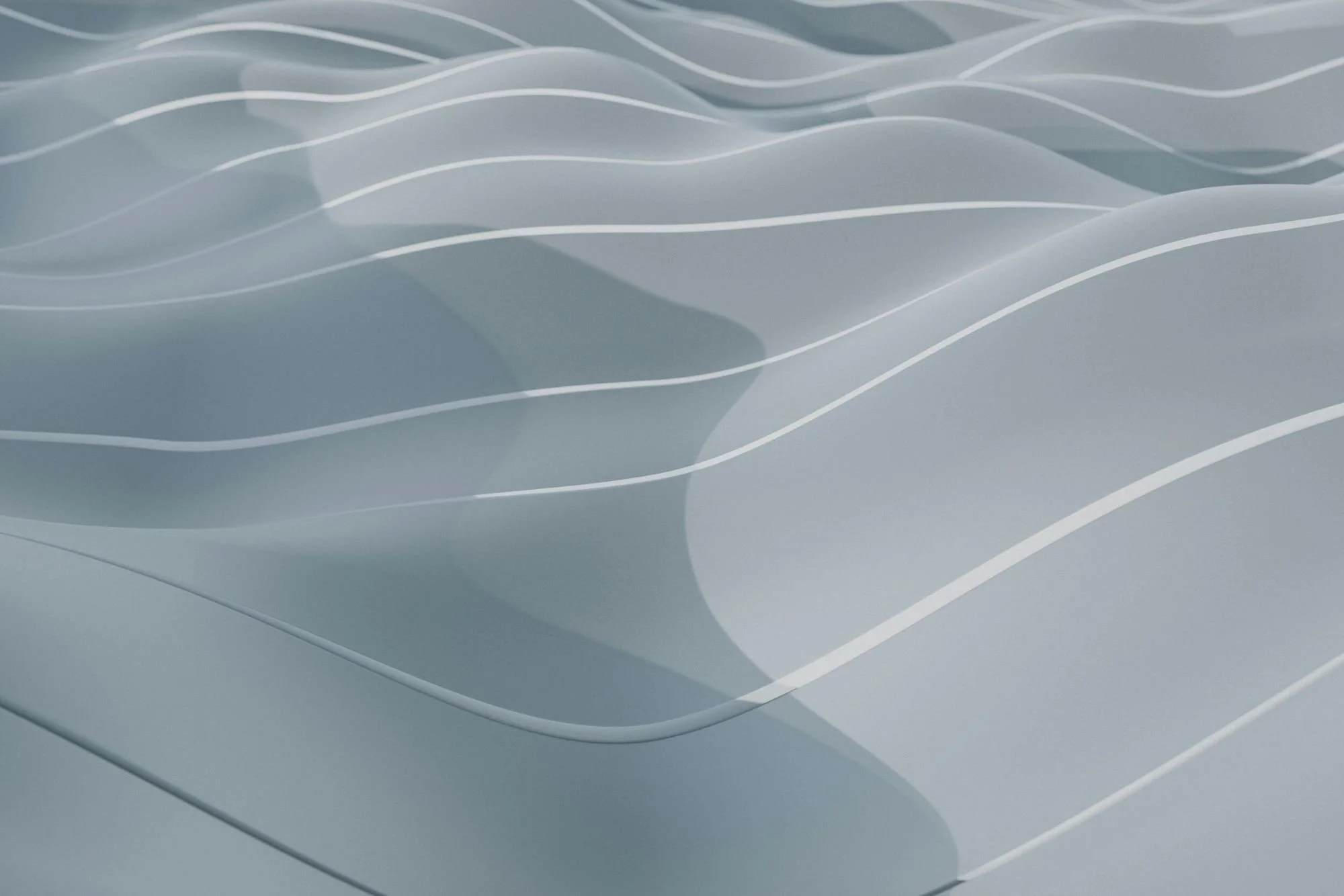
PhD Dissertation Abstract
PhD Dissertation Abstract
d-RBD Illustrated: PhD Dissertation Abstract
“Wind turbine foundations transfer dynamic and highly eccentric loads to variable soils. The design of such foundations involves the verification of multiple limit states to ensure proper operation of the turbine and avoid catastrophic failures. An optimal foundation design minimizes cost while meeting all relevant limit states at quantifiable and acceptable risks. This dissertation explores three common limit states that are relevant to the design of shallow, gravity based, wind turbine foundations using a fully-probabilistic Monte Carlo Simulation (MCS) method, termed in this work as the direct Reliability Based Design (d-RBD) method. The three limit states are foundation tilt, rotational stiffness and bearing capacity. For each of these limit states, design variables are randomized using predefined probability density functions. The d-RBD method involves running Monte Carlo Simulations to produce realizations covering potential combinations of design decision variables such as foundation dimensions (e.g. width and/or depth). The d-RBD method uses Bayesian conditional probability theory to select the geometry combinations that meet the predefined target probability of failure. With this approach, a single MCS run is needed to identify pools of acceptable designs for each limit state from which an optimal design meeting all limit states can be selected. This dissertation introduces d-RBD as a direct, fully probabilistic design procedure that offers important advantages over global factor (ASD/WSD) or partial factor (LSD/LRFD) design methods. For each of the limit states under consideration, d-RBD is used to highlight the cost of uncertainty, rank the design variables by their importance and assess the effects of pertinent variability assumptions. The findings from this work are relevant to ongoing efforts to develop international and U.S. standards for the design of wind turbine support structures and their foundations.”